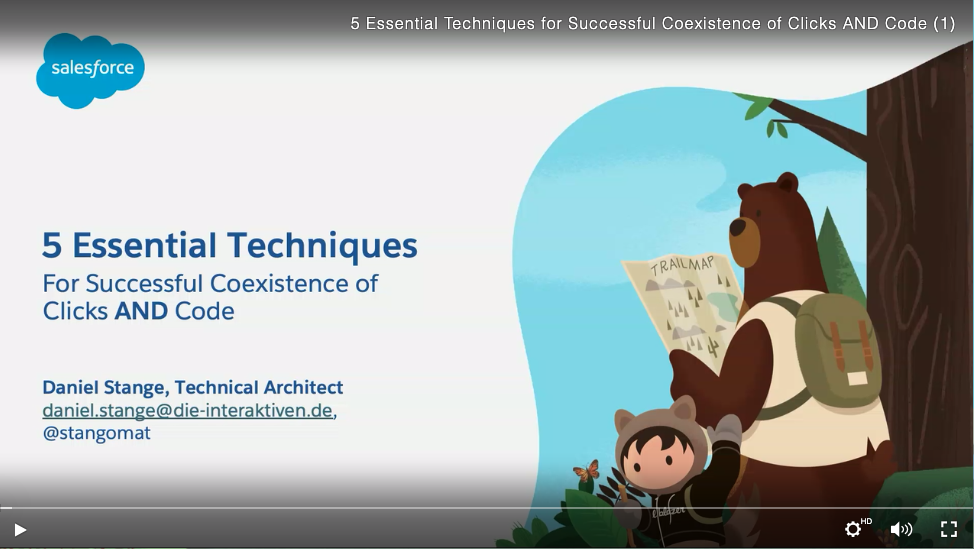
My Dreamforce presentation “5 essential techniques for successful coexistence of clicks AND code” is now available on Salesforce Videos (originally held Oct 20, 2019 and Oct 21, 2019. The recorded session took place on Oct 21, 2019.
Der ganze Gemischtwarenladen…
My Dreamforce presentation “5 essential techniques for successful coexistence of clicks AND code” is now available on Salesforce Videos (originally held Oct 20, 2019 and Oct 21, 2019. The recorded session took place on Oct 21, 2019.
In the past blogpost, we have prepared and upload an XML export from our Franke A400 coffee machine. As we’re working with machine data, the data prep journey isn’t over yet. Let’s talk about how to find out what’s in the data, what’s left to be done, and how to resolve these issues.
One nasty thing about working with machine data is, while typically very clean and structured (because written out by a machine following predefined rules), it’s not designed to be read and understood by humans in the first place. In order to work with this data, we have to take a deeper look at the data loaded to analytics and try to get to the meaning of most of the relevant fields.
Continue reading “Coffee Dashboard: Processing and Optimizing the Dataset”When you begin to build anything related to data, you have to get a clear understanding about the question(s) you want to get solved, and the data you want to use to “ask” to find an answer. What sounds like a banality is indeed an essential technique of breaking down questions on the one hand, understanding your data sources on the other hand, so that you eventually get to questions, data and granularity that matches so that you can finally start building.
Continue reading “Coffee Dashboard – Preparing the Data”When I started with Einstein Analytics, I had little business data at hand and I wanted to build something that I could demo to customers in a way that allows them to understand what Einstein Analytics is capable of in a friendly and entertaining way, but without the need of showing our company’s data or fictitious data that people can or cannot relate to. It was just the same time that we got a new, professional coffee maker that can export machine data that gives details on the coffee consumption.
Well – a coffee dashboard! Now that’s something everyone knows: isn’t the coffee maker the number one social space in every company? Isn’t great coffee more than a perk, but rather a necessity of work in the 21st century?
Long story short: I have built this dashboard. I have maintained it for a while, and it always served its purpose very well. And Einstein Analytics moves more and more to the center of our business intelligence, requiring me to take better care of the assets we hold in Analytics and especially decluttering our org from time to time. I removed the old coffee dashboard to keep the org clean, and this could have been the end of the story…
… until I posted about my success in passing the Einstein Analytics and Discovery Consultant certification. I mentioned the coffee dashboard and people did ask for that. So I decided to rebuild it, as it is a good tutorial on how to leverage the features of Analytics to consume external data, run some preparation and build a fancy and fun dashboard. So – join me on this ride back to my Einstein Analytics roots in what was then Wave Analytics. I plan to blog about each step on bi- or triweekly basis.
July has been really exciting so far as I finally managed to complete my Einstein Analytics and Discovery Consultant certification! I was provided with a free voucher that was only valid until July 31, so I really had to give it a shot and see how I did. Luckily enough, I knew enough to pass – and here’s how I did it.
Continue reading “Analytics certified… finally!”Another community conference debut in 2019 is CzechDreamin’ in Prague. Martin Humpolec and his team have crafted a wonderful four-track conference, and there’s plenty of interesting stuff to learn: Rikke Hovgaard will give an Analytics workshop, René Winkelmeyer and Philippe Özil will present on LWC, and much, much more. If your calendar allows, grab one of the last tickets and come to Prague on August 16th.
I’ll be hitting the stage twice:
Again – get your tickets while stocks last – it’s going to be the #1 summer conference in Europe!
Christian Szandor Knapp and I ran a hands-on workshop at the first installment of the YeurDreamin’ conference in Amsterdam on June 14th, 2019. It’s been a wonderful summer day, and the Amsterdam crew – Paul Ginsberg, Kathryn Chlosta, André van Kampen, Kevin Jackson, and their team – really made it stand out.
[slideshare id=158181315&doc=yeurdreamin19wow-flow-workshoppublic-190726195726]
Continue reading “YeurDreamin ’19: Put the Wow! into your Flow”I was a returning speaker at ForceAcademy LA on May 13, 2019, with a presentation on the relation between clicks and code – the things that can possibly go wrong and how to find and fix them.
Clicks AND code, limits, performance and the Order of Execution – all of these are key topics that I’m doing a lot of research on.
I was hitting the stage once again with Christian Szandor Knapp. This time, we were trying to make life better for Salesforce Admins. In our humble opinion, Salesforce DX and especially the CLI are the number power tool for admins – that’s what we promoted this idea at French Touch Dreamin’ in Paris on November 24th, 2019.
Video to follow later.
Resource: https://github.com/open-force/sfcli-cookbook